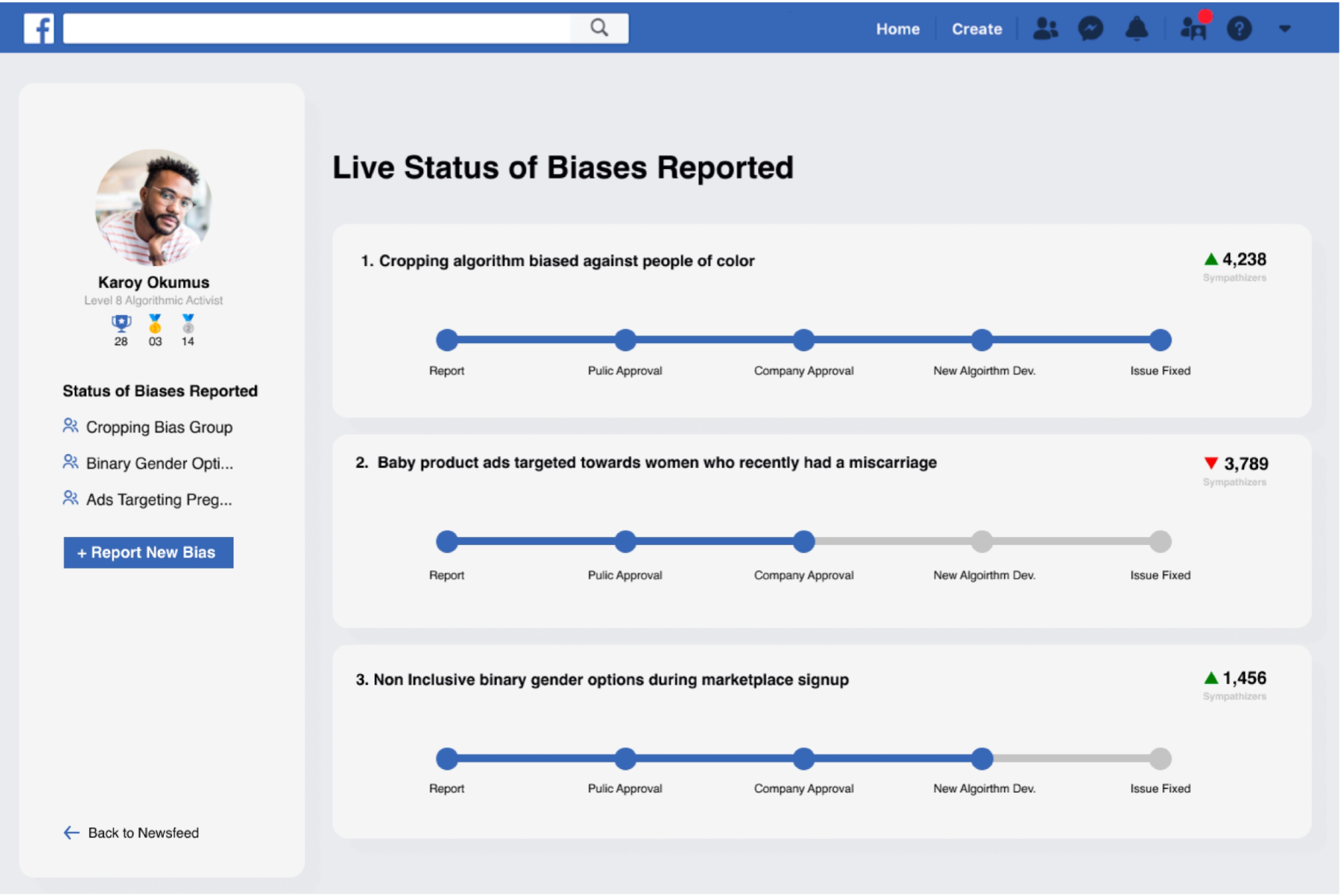
Algorithmic Activists
October - December 2021
Carnegie Melon University Coursework—Human Computer Interaction Department
User Centered Research and Evaluation
Skills used: semi-structured interviews, contextual interviews, think-aloud protocols, speed-dating, affinity diagraming, and pitched research
Project by Gabriel Alvarez, Healy Dwyer, Pradeep Nagireddi, and Harvey Zheng
Role: UX Researcher
As a UX Researcher, my goal was to learn about people's experience with algorithmic bias on social media, how community leaders organize their members and keep them motivated, as well as help brainstorm and recommend potential solutions to the problem.
More specifically, I conducted background research in the problem space, generative think aloud testing, contextual interviews in the form of directed storytelling, analyzed and synthesized our research data into insights through affinity diagramming, created storyboards, and used speed dating to find viable solutions.
Overview
When users encounter some kind of bias on the web, whether via an advertisement or a resume screening system, they may not know how to react. We created a recommended solution for social media companies to implement into their platforms involving a dashboard for users to track progress on reported biases, and a space for users affected by a bias to discuss potential actions or support each other.
This solution helps people make sure their voices are heard, talk with others who are affected by the problem, and see that their reporting made some kind of an impact so people continue to report problems while staying active on their social media platform.
The Problem
Many users will encounter algorithmic bias, but will not know what to do after encountering. As an example, when cropping for a preview in the past, Twitter would preview a white person's face rather than a black person's based on facial recognition, with the position not mattering. This issue went viral, which got Twitter engineers to notice.
Our task was to find ways to crowdsource the auditing of biases in algorithms, whether AI/ML based or programmed.
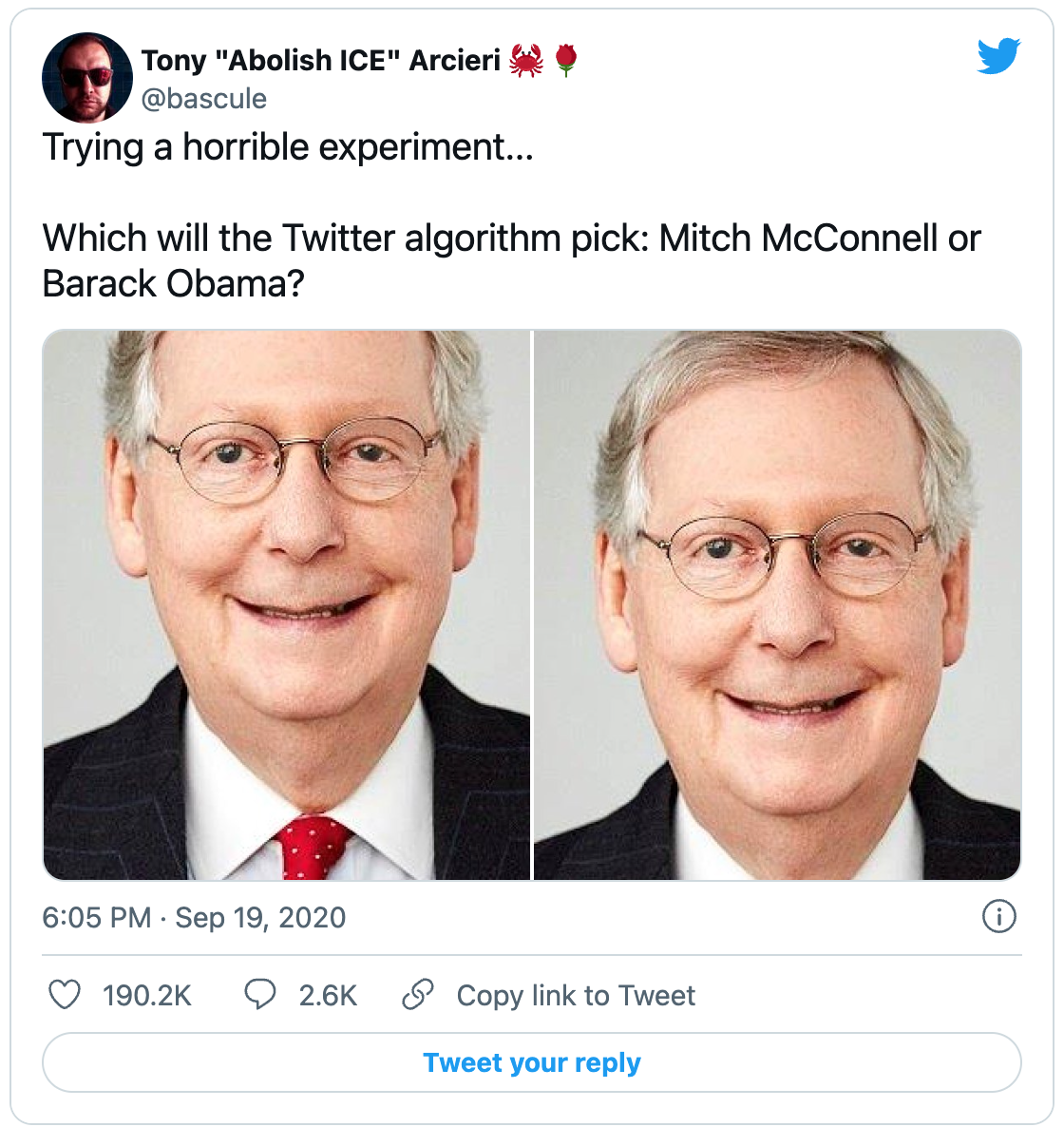


Research Process
Our research involved 12 interviews, using methods such as generative think aloud, directed storytelling, and speed dating to help us really get at the root of the problem, and how we might solve it.
The process started out with analyzing Tweets about three different algorithmic biases that arose on Twitter, conducting primary research by browsing social media sites for algorithmic bias, and doing general secondary research from academic and news articles.
We then transitioned into think aloud protocols, where we asked users a variety of questions about interacting on a community driven forum such as Reddit. With these think alouds, our main intention was to find why people became a part of certain communities: what might motivate them to interact? what might cause them to report something? who might they trust online? We mainly learned that people would interact in communities they had some kind of personal stake in, which included reporting problems. They would trust users who frequent the site, and who other users also seem to show trust to. From the think aloud, we created usability finding reports.
With these insights in mind, we conducted directed storytelling with community and organization leaders to learn more about how they manage communities; specifically, how they organize meetings and help people stay on task, how they create a good group dynamic, and what they may do if they see someone lose motivation. After doing interpretation sessions and synthesizing the research into an affinity diagram, we found that, again, people will participate in something if it's something they care a good deal about. We also found that being casual and personable to your community helps them become more comfortable. Lastly, if they see someone losing motivation, having a one-on-one conversation, rather than calling them out, is also a good idea.
Finally, after doing Crazy 8s to brainstorm solutions, we conducted speed dating on more community leaders to validate insights as needs, as well as to help generate potential useful solutions to the needs.
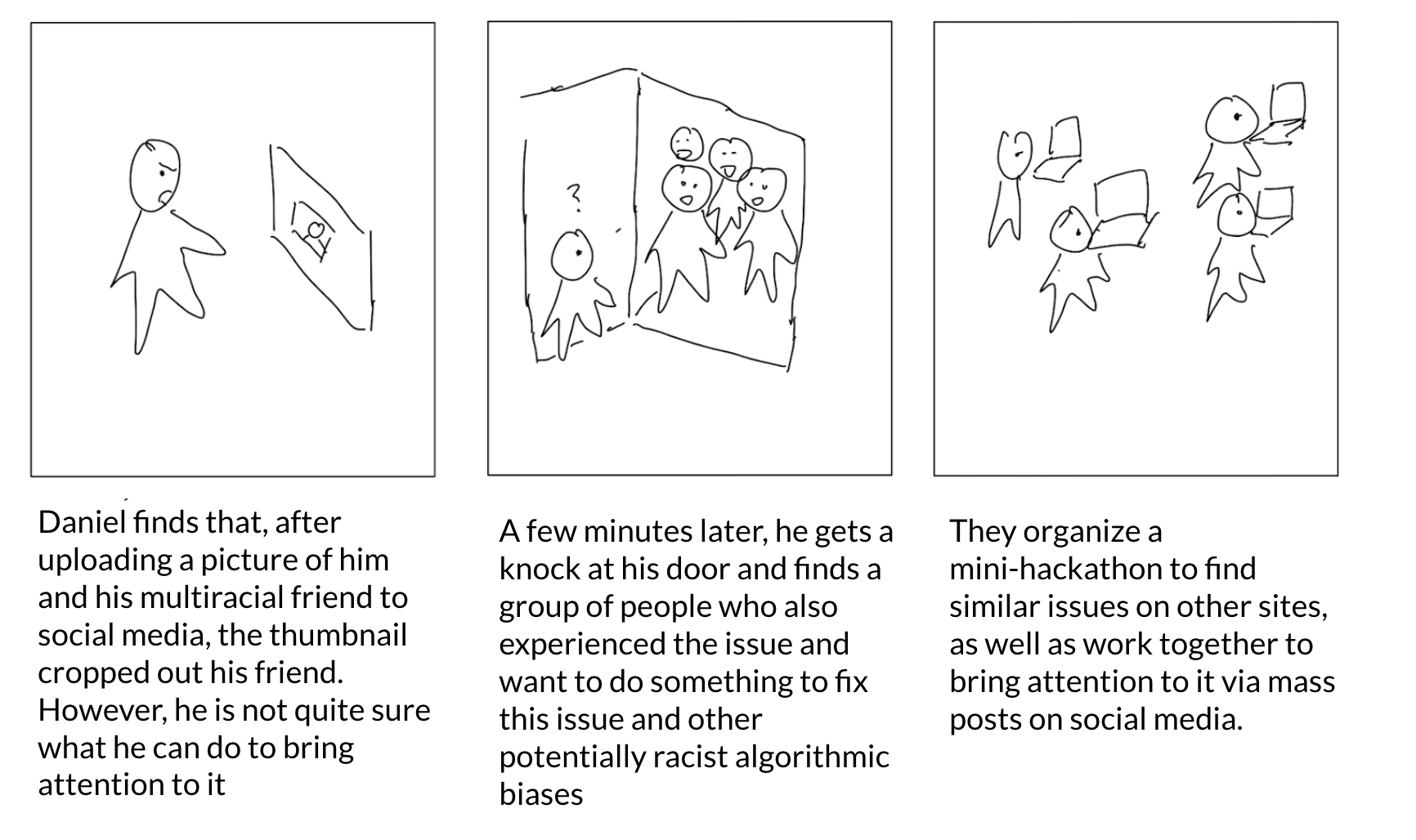
Example storyboard
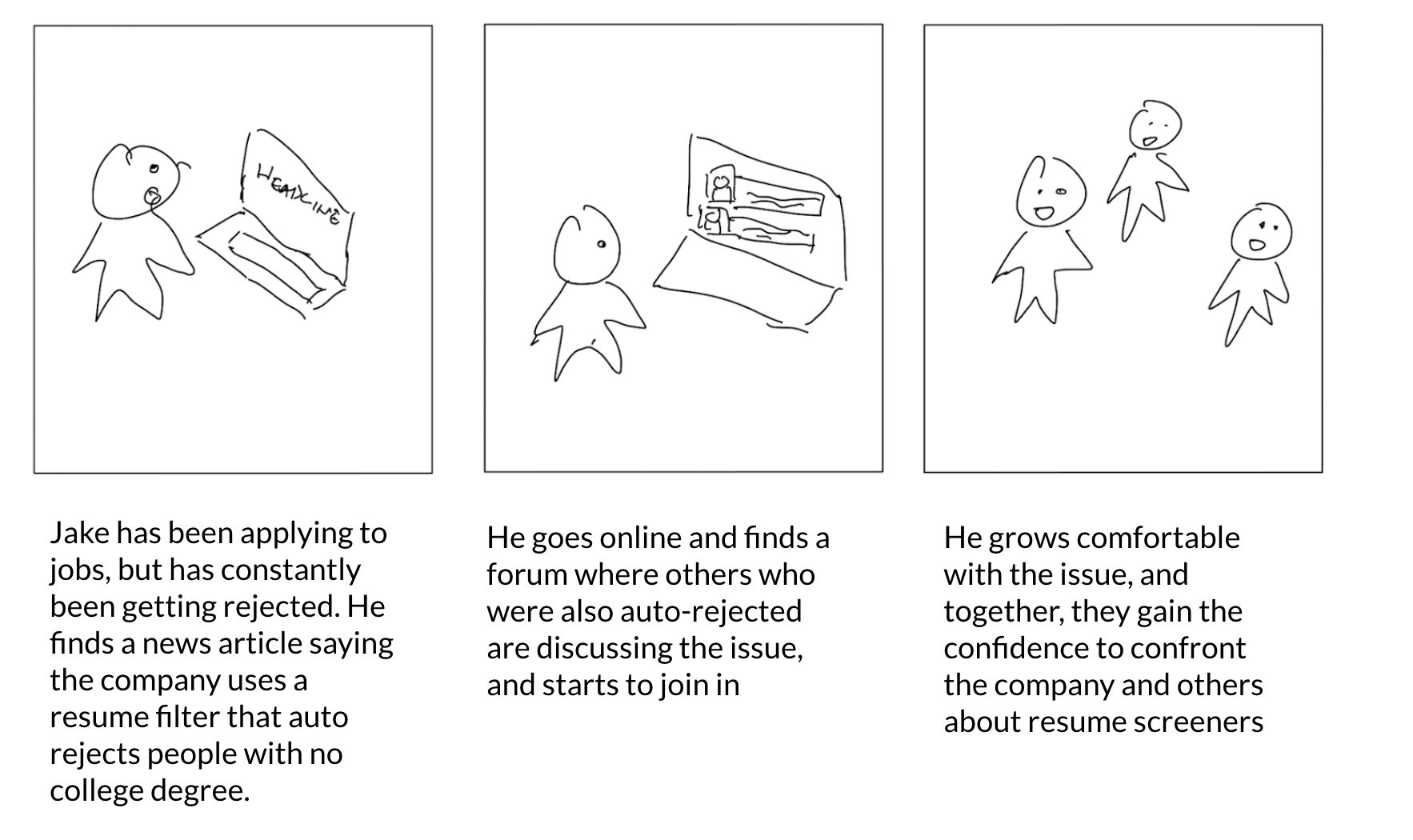
Example storyboard
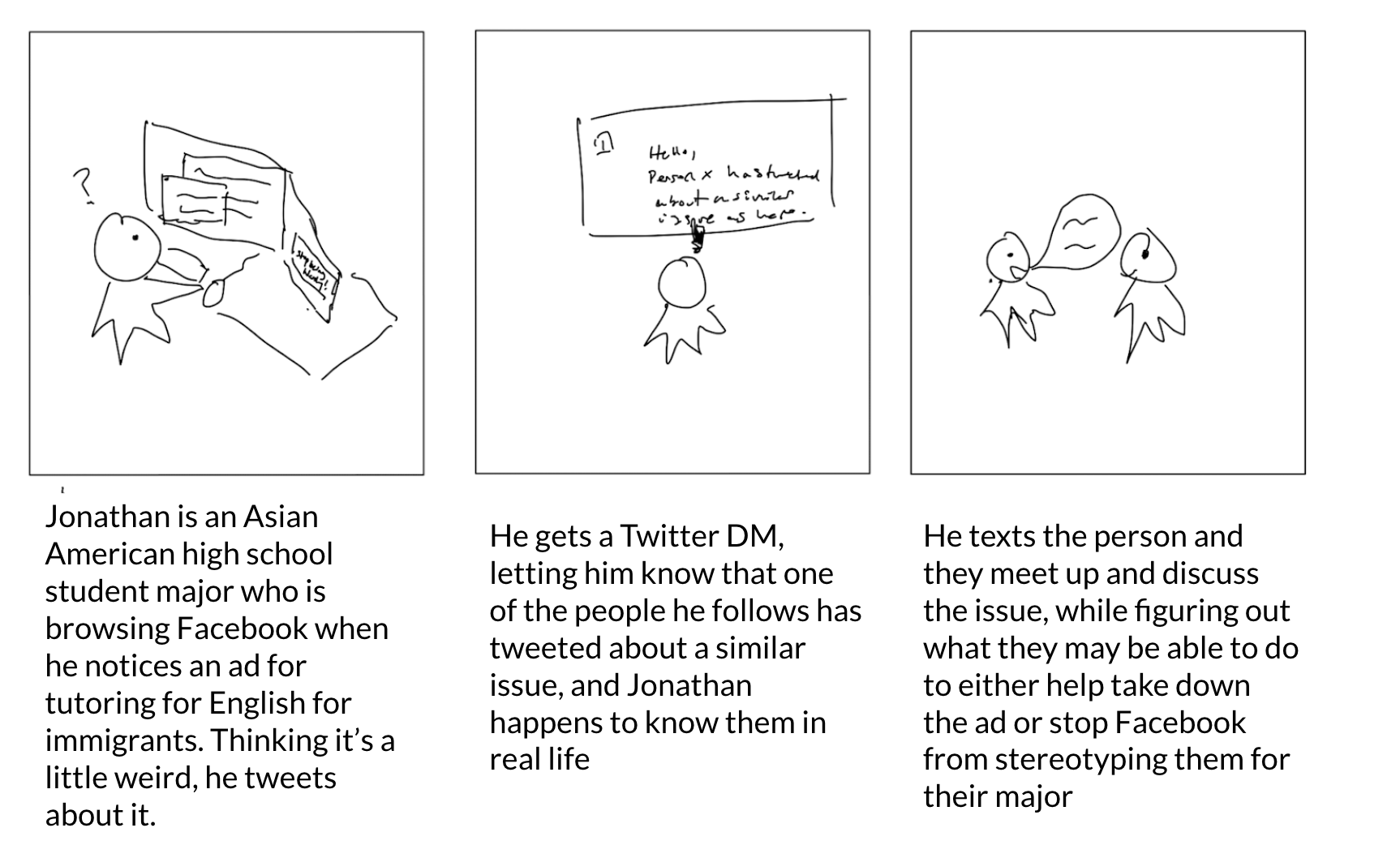
Example storyboard
Findings and Insights
From our research, we found five main insights into the issue of how to rally a community to report and become activists for a particular algorithmic bias.
First, we found that personal connection helps volunteers stick longer and contribute better to a cause. Throughout our three sets of interviews, this was a consistent trend—people would participate in something because being in the community meant something to them. As an example, in our think aloud testing, an interviewee mentioned they'd likely join subreddits for topics they're casually interested in.
Second, clear signs of progress and improvement help people stay motivated. Especially in areas with delayed gratification or progress, having some kind of feedback gave people the sense that they were making some kind of an impact. By seeing their progress, people are more inclined to keep going.
"I think big thing to make people be unmotivated is when you don’t see the direct result, especially when you are spending lot of time on them"
Healthy communities also have a common trait of assuring every member that their opinions matter and were heard. Through our interviews, we discovered that people struggled in communities where people didn't feel like they were being heard, and people thrived in places where everyone participated and was valued.
"I really think having a casual environment encourages more participation in discussions, for my [community] at least"
Our interviews also pointed to moderation being key to keeping community spaces safe. With no moderation, people can feeling less motivated to participate. Moderation helps build a safe space for people to communicate issues they might not be comfortable saying, and give people a support structure to lean on.
Finally, we found people feel isolated when encountering the online bias and need communities for support and boosting self esteem. In some communities, people will be anonymous and not really want to speak out to others, but instead lurk.
Solution
Our recommended solution includes two parts: a dashboard to see progress of reported biases, and a way for people who were affected by a bias to discuss the issue, and possibly organize around the issue to audit other sites. This could either be integrated into social media platforms, or developed as a third party platform, like GlassDoor or G2 might.
With this solution, we aimed to give people affected by algorithmic bias a way to collect themselves and organize action against a company and other social media platforms to get them to develop a solutoin. We also wanted to give people a way of seeing feedback, and knowing that their reporting made some kind of an impact.
Unfortunately, with the length of the semester, we did not have time to try developing or testing higher fidelity versions of our prototypes.
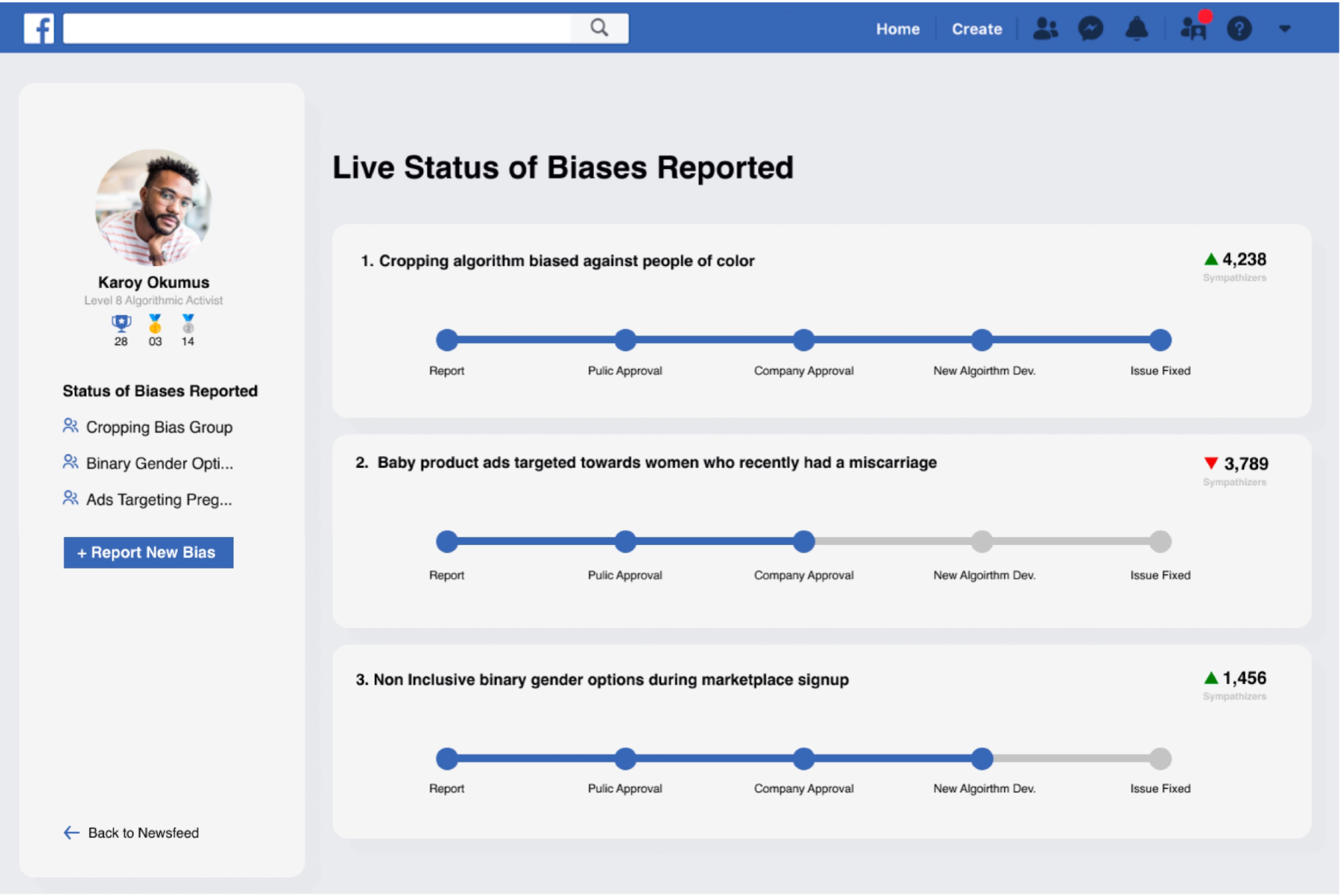
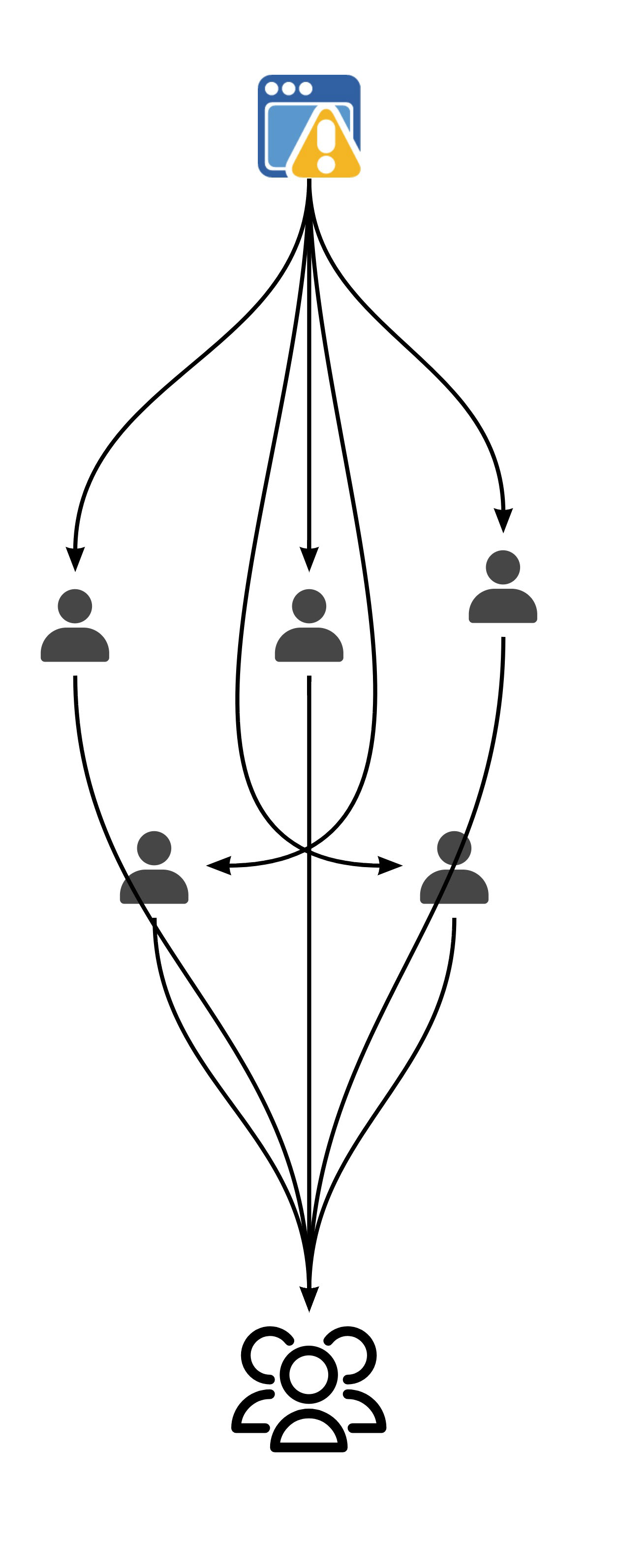
Future Considerations
For future steps, we would want to start out by finding a way to incorporate the dashboard for social media users that makes it intuitive for them, whether in a third party app or in-app. Next, we would want to ask social media companies the likelihood they would implement something like this, and figure out what would get them to implement our solution. Finally, we would want to test further, and then deploy.